What’s Big Data Analytics? Can you Use it in Marketing?
Big Data Analytics is a broad term, a big umbrella that contains other methodologies and tools, aiming to control all the data of your company and brand.
The issue is that this control is often hampered by the volume of data and the complexity of Analytics systems.
Power B.I., for example, has been and still is considered one of the best tools for Business Intelligence and Big Data.
But it is somewhat complicated to use for people who are not accustomed — or even specialists — in its functionalities.
However, Big Data Analytics is changing significantly. Today, with Generative AI, many advancements are being made to improve the accessibility of these tools.
Due to this greater democratization, today we need to talk about Big Data Analytics — taking the term down from the pedestal of specialization and bringing the conversation to everyone.
Let’s understand what Big Data Analytics is, its relationship with marketing, and what advancements AI is bringing to the field.
To start: What is Big Data?
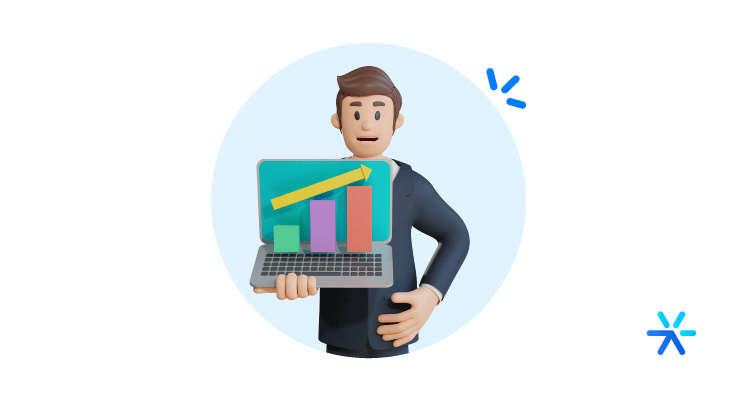
Just to contextualize: the concept of Big Data is not for all companies or all market segments.
Usually, companies dealing with some aspect of Big Data are larger, much larger, that need to handle a vast amount of information.
Or they don’t even need to deal with this vast amount of information: they seek large data sets to gain a greater competitive advantage.
The definition of Big Data is precisely this: data sets so large that they cannot be processed and organized in a common system, not even by analysts alone.
The concept of Big Data is closely related to Business Intelligence. IBM coined the term in the late 1950s, defining the activity as:
“The ability to understand the interrelation of presented facts to guide actions aiming at a determined objective” — IBM, see more in the History of Big Data.
Let’s go to a practical example? Before Web 2.0, which is the historical moment we are living in today, it was impossible to store detailed information about all site customers.
Simply due to a lack of data structuring. To simplify, it was as if each customer profile file was too large.
Handling these files becomes even more challenging considering huge e-commerce sites, which make tens of thousands of sales per month.
Starting in 2011 — it’s that recent! — a new framework emerged: Hadoop. It was open source, which allowed more research and developments in the area, culminating in the Business Intelligence software — including AI — that we have today.
Was it easy to understand what Big Data is? I hope so, but we still have a few more points to elucidate that will help you understand even more.
These are the three V’s of Big Data. After discussing them, we will be much better understood about what the concept really means.
After that, we will be able to move on to what Big Data Analytics is — and how to do it.
The Three V’s of Big Data
To understand what is truly considered Big Data, the three V’s serve as an excellent guiding tool.
These V’s serve to formalize what types of data are understood as Big Data and also give us the roadmap to start understanding how they are treated.
The three V’s of Big Data are:
- Volume: Big Data, as a concept and also applied as a strategy through Big Data Analytics, deals with an enormous amount of data. We gave the e-commerce example before, but we can think of others: history of interactions and mentions of your brand on Twitter, for example. Or clickstreams from a website, industrial sensor data, etc.
- Velocity: refers to the speed at which this data arrives. They need to be stored at the same speed they arrive to avoid bottlenecks in servers and systems. Usually, this V is related to storing information in local memory instead of the disk, which is a slower process. This is a very technical point.
- Variety: this is the big “catch” of Big Data: most data considered Big Data is unstructured — that is, they do not fit into existing frameworks, like Google Analytics, for example. Think articles, videos, audio, etc. Structured data would be “number of comments,” while what the comments are saying are unstructured data. Due to this characteristic, part of the work and difficulty of Big Data is pre-processing and creating new structures for this data.
➡️ Read also: Marketing Types - 39 Strategies for Your Company
So, do we understand what can be considered Big Data?
Basically, they are unstructured data, extremely varied, arriving at high speed and needing to be interpreted also at high speed, to ensure real-time monitoring on your Dashboard.
And of course: all in large volume.
That’s why we made it clear at the beginning of the article that not all companies will even come into contact with Big Data.
Most of the data captured in conventional digital marketing is structured data. And those that aren’t can be structured through Google Analytics events.
All clear so far? Then let’s move on to the main part of the article: what exactly is Big Data Analytics?
What is Big Data Analytics?
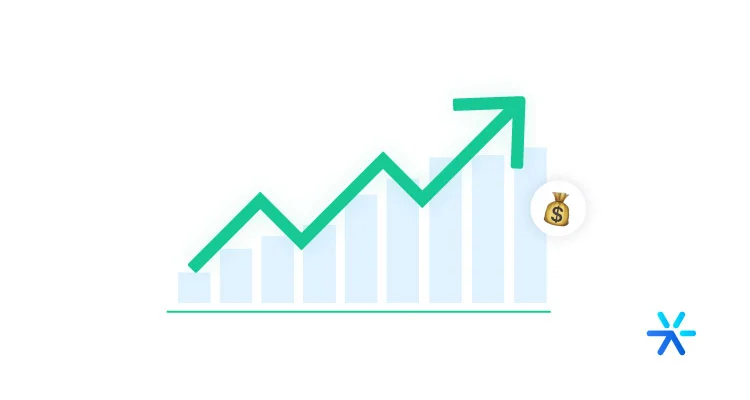
As you might imagine, Big Data Analytics is the work of capturing Big Data and analyzing it in the best possible way.
It is part of the work of Business Intelligence, which seeks to store and interpret this data to take actions that bring the brand closer to its business objectives.
The most important aspect of Big Data is, by far, the interpretation the data receives.
It’s only through these interpretations that we can use this knowledge for something that makes sense for the brand.
An example to make it clearer: a company decides to catalog all mentions of its name on Twitter.
🤓 Read also: Twitter Marketing: An All in One Guide
Throughout this topic, I’ve brought some items that will help us better understand what Big Data Analytics is and how this work is done.
Take a look:
What types of data does Big Data Analytics analyze?
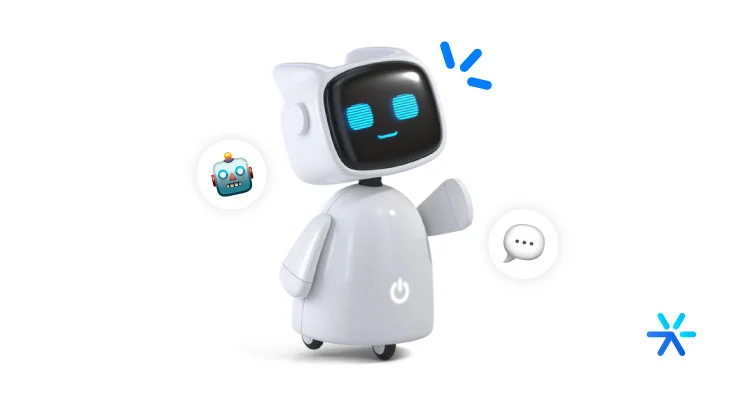
In the world of data analysis for digital marketing, there are three major categories:
- Structured data: that is captured to fit within a specific framework. The number of visitors to a website within Google Analytics, for example, is structured data because GA4 has a structure created to capture and store it. Or a list of qualified leads that you can export from one marketing automation software to another without problems.
- Semi-structured data: a website, for example, is semi-structured data. It can be analyzed within a framework, but to make this happen, the framework needs to be created first. They are structured because they follow a pattern — in the case of the website, the HTML, CSS, JavaScript, etc. pattern — but they do not follow a simple analysis pattern.
- Unstructured data: are data that are not in any framework nor follow a logic. Videos on TikTok, for example, only have their format in common. Comments on Instagram only have in common the fact that they are articles. The data is what the video says, what the comment expresses. These data are the most important for Big Data Analytics, but they are not structured and need to be processed by the Big Data framework — that is, they will be structured within the system.
How does Big Data Analytics Work?
Think of a simple computer, the laptop you’re using to read this article right now, for example.
You can open a spreadsheet with 20,000 cells without any problem. 40,000 cells are already a bit too much. 100,000 will cause slowness.
And this spreadsheet is just structured data, usually only text, raw information.
Now imagine that, at the same time, your laptop needs to organize this data and interpret all the unstructured data — videos, comments, etc.
You would need a NASA PC to do that. The operation of Big Data Analytics — here considering both the concept and the hardware and software — aims precisely to allow this analysis to happen.
This occurs in four distinct stages:
Capture:
The Big Data Analytics software must be capable of capturing the information. This is often done through API integration. This data is then stored in a repository for processing.
Processing:
The information is then processed and filtered, seeking to facilitate the integration of this data into the Big Data framework used by the software.
Cleaning:
The data is then “cleaned” — duplicated, irrelevant information, etc., is checked and deleted.
Analysis:
The software then seeks to extract insights from the obtained information and present them to the users in the best possible way. This is where it becomes possible to create charts, reports, etc.
For those who understand English, it’s worth reading this article from Microsoft, detailing all the technical aspects — from database frameworks to the presentation of information — behind Big Data.
What are the benefits of Big Data Analytics? And its challenges?
The biggest benefit of Big Data Analytics is the ability to analyze data that, before these specific frameworks, simply could not be analyzed at all.
Analyzing this data is fundamental for large companies, which can achieve results in various areas, far beyond profits or losses.
See an application in the Public Relations area, for example. A company that uses Big Data Analytics can easily measure its reputation by analyzing comments from a social network, for example.
Or from all social networks at the same time.
The biggest challenges of Big Data Analytics, however, are mainly related to its complexity.
Today, dealing with Big Data Analytics systems is a complicated task, primarily intended for software specialists.
Companies hire people with this specific knowledge to operate these systems. And for many years, this was the only way to access everything Big Data Analytics offers.
However, this has been changing gradually, mainly with AI. We will talk more about this throughout the article.
But first, we need to delve even deeper into the practical part of Big Data Analytics. Come with me:
Possible applications of Big Data Analytics in the industry
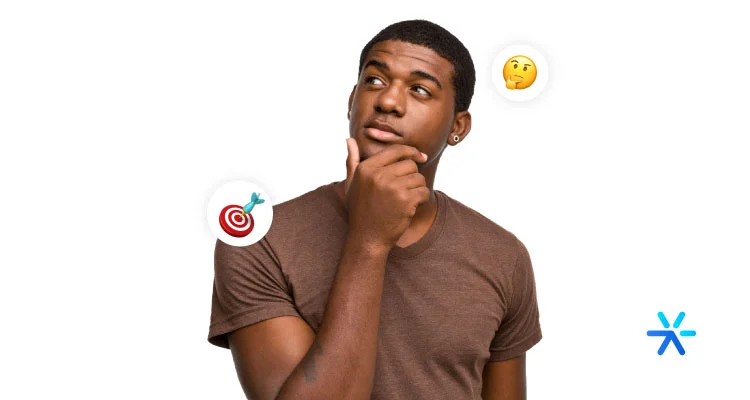
The applications of Big Data Analytics are quite varied.
Many of them are related to Business Intelligence. Throughout the article, all the practical examples we brought are entirely related to this purpose.
However, it goes well beyond that.
I have separated in the next items some more out-of-the-box use cases for us to better understand all the possibilities of Big Data Analytics.
Come with me:
Sensors and industrial automation
Large industries deal with an enormous amount of information in their machinery.
A single machine alone produces a vast amount of information all the time.
And specific machines produce specific information that needs to be cataloged and analyzed.
These data range from structured to unstructured and are arriving at the system all the time.
The only way to categorize and store everything is through Big Data Analytics.
Even more so when industrial automation comes into play. In this case, collecting this information goes beyond compliance: for the system to function, it is necessary to operate with Big Data.
Business Intelligence
B.I. is about data integration. It is about finding correlations between data and applying them in a way that makes sense for a company’s business objectives.
A quick example: the public’s reaction to the announcement of a new acquisition can indicate a drop or rise in the stock value of the two companies.
Stocks, as we know, have their value dictated by the opinion of the public that buys them. So, understanding the reaction in real-time, it is possible to prepare for times of devaluation or increase the advantages when appreciation occurs.
Business Intelligence goes far beyond that. As there is no need to work with structured data, any type of data can be stored and interpreted by these softwares.
Logistics, for example. Before launching an aggressive marketing campaign, it is possible to track in a few clicks the biggest complaints about deliveries in the last month to ensure a better experience for new buyers.
The possibilities of Big Data Analytics for B.I. are immense. That is precisely why these systems are usually created for this functionality.
But we still have another practical use before moving on to the next part of the article. But this one deserves a special topic. Follow me:
Big Data Analytics in marketing: Is it possible?
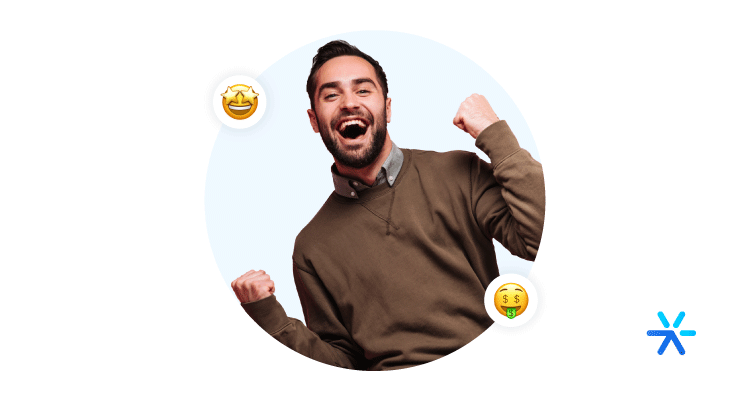
Yes, Big Data Analytics in marketing is quite possible. But with a caveat: it is usually applied by very large companies.
Small and medium-sized companies, in fact, do not even need Big Data Analytics in their digital marketing strategies.
They usually use Inbound Marketing, along with Content Marketing, for demand generation and lead generation.
The point is that these techniques generally deal with structured data. There is no need to go much beyond them for these purposes in digital marketing.
But the companies that go beyond this usually achieve great results with Big Data Analytics. Where exactly? Let’s find out together now:
Social media analysis
One of the ways Big Data Analytics is most used is through content analysis on social media.
This work is quite interesting. Using Natural Language Processing — not exactly NLP AIs, just the concept — Big Data Analytics systems can measure several points from what people say on social media.
The most obvious are brand mentions. Big Data systems can store all mentions of the brand and go a bit beyond just storing them.
With NLP, it is possible to determine very specific parameters about what is being measured. For example: it is possible to understand what percentage of mentions are positive and negative.
Moreover, it is also possible to make this association of positive and negative for any topic, informing the company about new trends that are worth addressing or not.
It is possible to integrate all this into your database to make it easier to reach marketing conclusions and launch new actions.
But the work of Big Data Analytics does not stop there:
Personalization in marketing
Big Data Analytics systems also allow for much greater personalization in your marketing.
Through these systems, it is possible to integrate other databases, specialized in customer reviews, for example, to identify the best qualities and biggest defects of a product.
And even before the product itself is launched: it is possible to understand positive and negative points of your marketing campaigns and your competitors’ campaigns.
And speaking of which:
Competitor analysis
Just as you can analyze your own results and mentions, you can also do the same with your competitors.
You can know what the predominant opinion is about each of your main competitors in the market and evaluate the level of competitiveness that your segment presents.
We have an article that talks a bit more about competitor analysis. Read more below:
Marketing research
Another way to use Big Data is to assist in conducting research.
All the parameters we discussed so far can inform the brand about some aspect of its target audience that they do not yet know.
For example: what day does your target audience usually go out the most?
With Big Data Analytics, it is possible to integrate social media as a source of information and obtain this knowledge.
But of course: all this is very specialized work that, in addition to software, also requires people to run them.
Examples of Big Data Analytics Usage by Major Brands
Now that we’ve discussed the possibilities of Big Data Analytics, it’s also a good idea to talk about the companies that are already doing this work.
How do these giants operate their Big Data Analytics systems? What are their most common uses?
By analyzing the examples below, it becomes clear that most large companies that need Big Data Analytics analyze their own data, as they produce it in extraordinarily large quantities.
This is the most common use of Big Data Analytics: understanding the behavior of the customers and users you already have.
Let’s go through some examples that will make all this much clearer. Follow me:
Amazon
Amazon has a gigantic global database of everything its customers do.
And the possibilities for interaction between the customer and the brand are enormous. Here are a few:
- Adding a product to the cart
- Completing a purchase
- Abandoning the cart
- Searching for various brands
- Leaving reviews
- Leaving photos and videos
- Making evaluations
Among many other points. Now think: all this information is added to Amazon every day, by the millions.
It is only through Big Data Analytics that Amazon can understand what its customers think of the experience.
And this experience includes everything: from the first search on Google to the moment the purchase arrives and beyond.
The only way to measure so many things at the same time is through Big Data Analytics.
Nubank
Nubank, like many other banks, uses Big Data Analytics to understand both the behavior of its customers and to perform advanced credit analyses and operate its anti-fraud system.
Card operators are always walking a tightrope. The risks of operation are immense, ranging from fraud to default.
A Big Data Analytics system helps Nubank to discover new business opportunities while keeping the operation running smoothly, ensuring more leeway for its investments.
Netflix
Each Netflix user generates an enormous amount of data every time they access the platform.
What movies they watched, what movies they liked, what titles they played and stopped, how long they watched, and so on.
The best way to personalize these users’ experience in real-time is through Big Data Analytics.
In fact, what we understand as the “Netflix algorithm” is precisely this: an automated interpretation of the user’s tastes offering personalized suggestions, also through automation.
But Netflix goes further. How does it know, for example, which series is trending on the platform today?
By knowing this information, Netflix can determine which titles deserve renewal and which will be canceled.
And Big Data Analytics even extends to marketing: with in-depth social media analyses, Netflix can understand not only which series are the most popular but also which characters within these series are the audience’s favorites.
With this, new actions are imagined within the marketing universe of the streaming giant.
The Best Big Data Analytics Tools
Well, we understand almost everything there is to understand within the Big Data Analytics scenario.
What’s really missing for us to discuss are the tools that make the work possible.
Working with Big Data Analytics means working with software. It is not possible to work any other way.
But what are the most used software? I made a quick compilation here with the best tools on the market.
There isn’t much to say about them other than that they are the main ones when it comes to Big Data Analytics.
In the end, all offer similar services: the ability to perform Big Data analyses customized for your company.
The main Big Data Analytics tools on the market today are:
- Apache Hadoop (the original)
- Cloudera
- Tableau
- MongoDB (an open-source framework, similar to Hadoop)
- IBM Cognos and Watson
- Microsoft Power B.I.
So, is it clear what Big Data Analytics is and what its main applications are for marketing and Business Intelligence?
Now it’s time to start generating some data to your business.
Start with the basics: first-party data. You can capture it with our chatbot easily – just set it up and create a couple of questions. Done!
Try out Leadster for free right now – just click on this link.